What is Augmented Analytics in Data Science
Last Updated : 01 May, 2025
Data is god for companies these days! In fact, most, if not all, companies use data to analyze the current market trends, understand their customer requirements, and create their future corporate strategies. However, large multinational corporations definitely have an edge over small and medium-sized companies in extracting insights from data. Smaller companies do not have skilled data scientists or adequate resources to convert their data into meaningful analysis. In such a situation, their data is worthless for them if they cannot unlock its potential. But Augmented Analytics might change the situation! It may help in creating a more equal data-based corporate culture where all companies would benefit.
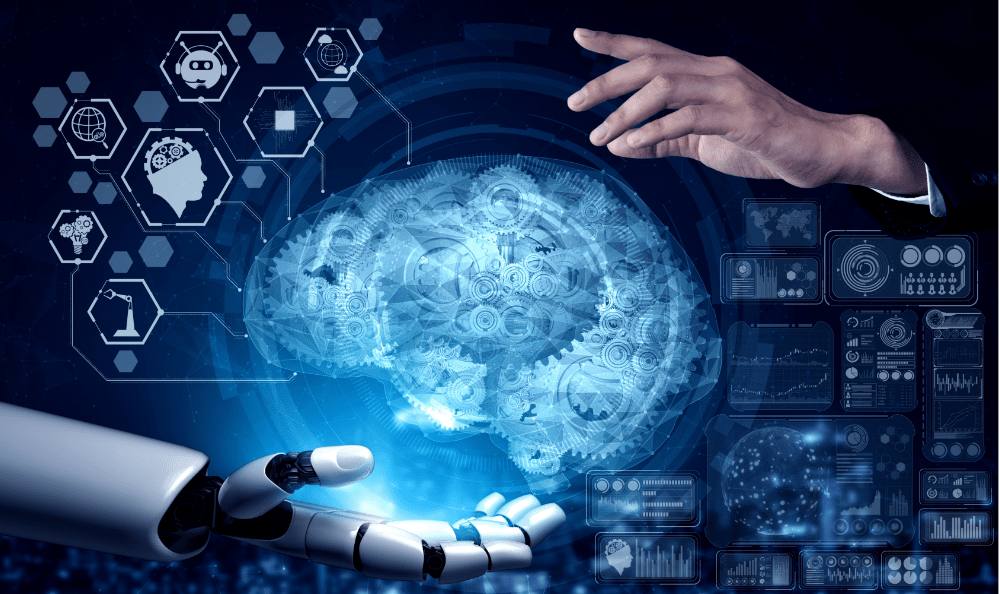
What is Augmented Analytics?
Augment analytics is a term that was originally created by the research firm Gartner in 2017. They claimed that it would be the “future of data analytics” and it sure looks like it! Augmented analytics basically uses Machine Learning and Artificial Intelligence to enhance data analytics by finding a new method of creating, developing, and sharing data analytics. The usage of augmented analytics in the industry means that companies can automate many analytics capabilities such as the creation, analysis, and building of data models. Augmented analytics also ensures that it is much easier to interact with and explain the data insights generated which help in data exploration and analysis.
Augmented analytics has also changed the entire working models for business intelligence. The addition of machine learning, natural language processing, etc. to data science has ensured that users can easily obtain the data, clean it and then find correlations in the data as the artificial intelligence will perform much of the tasks. Moreover, the AI will create data visualizations that will allow human users to easily find data relationships by closely observing these visualizations.
This is especially helpful in this current data age where there is a need to extract actionable insights from the data but not that many qualified data scientists are available. And even the data scientists don’t always have the business sense to determine the business actions that need o to be taken by seeing the data insights. So, Augmented Analytics is a godsend for many companies, and using it, their business professionals can also find insights from the data even if they have a little knowledge of data science and are not experts. Augmented Analytics has simplified Business Intelligence and allowed many smaller companies that are not data science giants to extract insights from their data.
Applications of Augmented Analytics
Augmented analytics can contribute a lot across the field of data science. It is especially changing the way Business Intelligence works in the tech industry. So let’s check out some ways in which Augmented analytics is contributing to the industry.
1. Automation in the Data Analytics Process
Machine Learning and Artificial Intelligence can contribute greatly to making Data Analytics a much faster process. When a data analyst needs to obtain insights from data, then Machine Learning can be used for automating all data processes starting from data cleaning and preparation, pattern identification in data, data visualization, creating auto-generated code, creating suggesting for data insights, etc. This will lead to a much faster data analytics process from start to finish.
2. Data Insights According to Context
Machine Learning can help Data Analysts to find new correlations and patterns in the data that they would have never found by themselves. So ML algorithms can take into account the context in which a data analyst is searching the data and help in obtaining data insights that are related to that context.
3. Conversational Analytics
Data Analysts can use Machine Learning and Artificial Intelligence along with Data Science for Conversational Analytics. This means that data users of various skill levels can access the data and obtain insights without being expert data scientists. They just need to ask questions from the data in natural language and the combination of ML and AI will allow them to obtain answers from the data in the form of charts, graphs, etc. and output information also in natural language.
Advantages of Augmented Analytics
1. Professionals can obtain faster data insights
When machine learning and artificial intelligence are used together with data analytics, that naturally results in faster data insights than normal. Data analysts can get cleaned and ordered data faster, accelerated data insights, and speedier data visualizations, which all lead to increased data productivity. Machine learning algorithms can automatically clean data which was done manually earlier, provide recommendations for patterns in the data which analysts had to find earlier, and so on. Moreover, data visualization tools use easy drag-and-drop methods for charts and graphs. All this makes data analysis much easier and faster.
2. Help reveal previously hidden data Insights
Earlier, data analysts needed an idea about what insights they wanted to discover from the data so that they could perform data analysis in a methodical manner to obtain those insights. But now, machine learning algorithms can be used to suggest patterns and correlations in the data depending on the insights required. In fact, using Augmented Analytics, data analysts can sometimes obtain surprising insights from the data that they never thought were there. This is simply because algorithms have no pre-created notions or biases about the data and they can find links and outliers in the data that humans would have not even considered.
3. Increases data literacy in smaller companies
This is the age of data and all businesses, big and small, are collecting large amounts of data. So, it’s important that all businesses are able to extract value from that data in order to perform better in this market. However, this was very difficult for smaller companies that did not have access to experienced data scientists. However, Augmented Analytics have evened the scale a little. Now normal data users without much experience of Data Science can find insights from the data with the help of Machine Learning and Artificial Intelligence. This has resulted in data literacy in smaller companies and the emergence of more widespread Business Intelligence tools.
4. Increases the user trust in data
Data analysts or data users work together with ML and AI algorithms to obtain insights from the data. These algorithms keep learning and they can guess the overall intent and business requirements of the analysts over time and provide data insights that are in line with these requirements. This increases the trust of the data users on the ML algorithms over time and so they can work seamlessly with them over the years. This unique trust is different for individual data users with the ML algorithms as for different companies and users, the business and user requirements from the data are also different.
Disadvantages of Augmented Analytics
Augmented Analytics uses machine learning and artificial intelligence algorithms to obtain results from data. However, if these algorithms are not trained correctly, then relevant information can be attained by the data analysis which is not useful for the company. So, it is very important that correct data should be used and the ML algorithms should be provided clear instructions to obtain useful insights.
2. Difficult to Scale Up
Augmented Analytics can require a lot of computing power as it uses machine learning and artificial intelligence to enhance data analytics. Moreover, it is very difficult to scale up as the increased volume of data along with ML algorithms to analyze that data can take too much time which is not feasible in a business setting.
3. Data Bias May Occur
Sometimes data bias can occur when data is biased and then machine learning algorithms are trained using this biased data. There is a risk in Augmented Analytics that this Data Bias will not be easily caught and the data insights obtained by the company are biased. So it is very important to have a check on all machine learning algorithms and also analyze the data thoroughly so there is no bias.
4. Quality Data is Required
Data can many impurities and inconsistencies but it is very important that quality data is used in Augmented Analytics otherwise the insights obtained will be useless. So the data should be cleaned and organized before any analytics is done to ensure that it is of top quality.
Conclusion
Augmented Analytics is changing the game for companies, especially smaller ones that lack expert data teams. By using AI and Machine Learning, it simplifies the process of analyzing data and finding insights, making it easier and faster—even for non-technical users. It helps uncover patterns that were hard to detect before, boosts data literacy, and allows users to interact with data in everyday language. However, like any technology, it has challenges such as the risk of data bias, the need for quality data, and high resource demands. Still, when used correctly, Augmented Analytics can empower all businesses to make smarter, data-driven decisions.
Similar Reads
What is Prescriptive Analytics in Data Science?
It can be determined that the purpose of any type of analytical service in the business field is to accumulate a huge stack of internally sourced data from public and other third-party resources into responsive feed to improve community operations. Prescriptive Analytics Prescriptive Analytics is th
6 min read
Data Science vs Data Analytics
In this article, we will discuss the differences between the two most demanded fields in Artificial intelligence that is data science, and data analytics. What is Data Science Data Science is a field that deals with extracting meaningful information and insights by applying various algorithms prepro
3 min read
What is Data Analytics?
Data analytics, also known as data analysis, is a crucial component of modern business operations. It involves examining datasets to uncover useful information that can be used to make informed decisions. This process is used across industries to optimize performance, improve decision-making, and ga
9 min read
Why Data Visualization Matters in Data Analytics
What if you wanted to know the number of movies produced in the world per year in different countries? You could always read this data in the form of black and white text written on multiple pages. Or you could have a colorful bar chart that would immediately tell you which countries are producing m
7 min read
Top 7 Applications of Data Science in Business
Data Science is a sub-part of Big Data that has characterized itself by velocity (of datasets reached unprecedented heights), variety (of datasets that can be collected and delivered in a single format), and volume (of datasets which are increasing massively with changing times). This lets businesse
8 min read
Top Applications of Data Science in E-commerce
One good thing because of the internet is the emergence of E-commerce websites. Now you can sit at home and order whatever you want, which will be delivered to your door! Want a new phone? Order it online! Want new shoes? Just type in your size and get them delivered! You can even order vegetables f
7 min read
What is Data Analysis?
Data analysis refers to the practice of examining datasets to draw conclusions about the information they contain. It involves organizing, cleaning, and studying the data to understand patterns or trends. Data analysis helps to answer questions like "What is happening" or "Why is this happening". Or
6 min read
What is Digital Analytics
The world is being transformed a lot to digitalize all the things in every field as the data is been freely flowing on the internet and it helps in making things easier. Data has now become a hot cake which helped many companies to get through and make use of huge data to improve their businesses. I
8 min read
What other than Python and R for Data Analysis
Big data have swept over the analytical science sector in MNCs and other corporates. A giant responsibility for mathematicians and analytics with Big data appears to make sense of the quadrillion data dumps obtained, thankfully there are many softwareâs that help them in this task. Python and R are
5 min read
Why Cloud Computing is Important in Data Science
Imagine a small company that wants to use data analytics to improve its services and gain an edge over its competitors. This company generates some data but it also accesses data from third-party sources to obtain insights. But the question is how to take advantage of this data? After all, this smal
6 min read