Receiver Operating Characteristic (ROC) with Cross Validation in Scikit Learn
Last Updated : 26 Apr, 2025
In this article, we will implement ROC with Cross-Validation in Scikit Learn. Before we jump into the code, let’s first understand why we need ROC curve and Cross-Validation in Machine Learning model predictions.
Receiver Operating Characteristic Curve (ROC Curve)
To understand the ROC curve one must be familiar with terminologies such as True Positive, False Positive, True Negative, and False Negative. ROC curve is a pictorial or graphical plot that indicates a False Positive vs True Positive relation, where False Positive is on the X axis and True Positive is on the Y axis. In this context, the False Positive rate is denoted as Specificity and the True Positive rate is denoted as Sensitivity.
Sensitivity = TP/(TP+FN) Specificity = TN/(TN+FP)
The top left corner of the ROC curve denotes the ideal point, where the False Positive Rate is 0 and the True Positive Rate is 1. You don’t usually get 1, but a score close to 1 is considered to be a good score.
ROC curve can be used as evaluation metrics for the Classification based model. It works well when the target classification is Binary.
Cross Validation
In Machine Learning splitting the dataset into training and testing might be troublesome sometimes. Cross Validation is a technique using which we select the batches of the different training sets and fit them into the model. This in return helps in generalizing the model and is less prone to overfitting. The commonly used Cross Validation methods are KFold, StratifiedKFold, RepeatedKFold, LeaveOneGroupOut, and GroupKFold.
We shall now implement the cross-validation technique to understand the ROC curve on different samples of the dataset.
Receiver Operating Characteristic (ROC) with Cross-Validation in Scikit Learn
Before we proceed to implement the code, make sure you have downloaded the sklearn Python module.
pip install -U scikit-learn
Import the required libraries
Here we will import some useful Python libraries like NumPy, Matplotlib, SKlearn for performing complex computational tasks in a few lines of code.
Python3
import numpy as np
import matplotlib.pyplot as plt
from sklearn import datasets
from sklearn.metrics import roc_curve, auc,roc_auc_score
from sklearn.metrics import RocCurveDisplay
from sklearn.model_selection import train_test_split
from sklearn.linear_model import LogisticRegression
from sklearn.model_selection import KFold
|
Read the Data
SKlearn provides various toy datasets from which we are loading breast_cancer dataset for our article.
Python3
data = datasets.load_breast_cancer()
X = data.data
y = data.target
print (X.shape)
print (y.shape)
|
Output:
(569, 30) (569,)
Define The Cross Validation and Model
In our case, we shall use KFold cross-validation and Logistic Regression since the data end target is Binary Classification.
Python3
cross_val = KFold(n_splits = 6 , random_state = 42 , shuffle = True )
model = LogisticRegression()
|
Initialize True Positive Rate and Area Under Curve
Since we are using Cross Validation, we will have different samples of training sets. So we will define the mean False Positive rate, True Positive Rate, and Area under Curve as a list or array.
Python3
tprs, aucs = [], []
mean_fpr = np.linspace( 0 , 1 , 100 )
|
Plot ROC Curve for every Cross Validation Split
Sklearn provides ROC Curve display metrics that take in the model and testing data as the argument to calculate the ROC curve on the given dataset. True positive and Area Under curve is updated on each split.
Python3
fig, ax = plt.subplots()
for index, (train, test) in enumerate (cross_val.split(X, y)):
model.fit(X[train], y[train])
plot = RocCurveDisplay.from_estimator(
model, X[test], y[test],
name = "ROC fold {}" . format (index),
ax = ax,
)
interp_tpr = np.interp(mean_fpr, plot.fpr, plot.tpr)
interp_tpr[ 0 ] = 0.0
tprs.append(interp_tpr)
aucs.append(plot.roc_auc)
ax. set (
xlim = [ - 0.05 , 1.05 ],
ylim = [ - 0.05 , 1.05 ],
title = "Receiver operating characteristic with CV" ,
)
plt.savefig( "roc_cv.jpeg" )
|
Output:
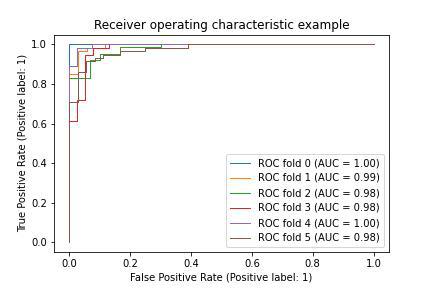
Similar Reads
Multiclass Receiver Operating Characteristic (roc) in Scikit Learn
The ROC curve is used to measure the performance of classification models. It shows the relationship between the true positive rate and the false positive rate. The ROC curve is used to compute the AUC score. The value of the AUC score ranges from 0 to 1. The higher the AUC score, the better the mod
4 min read
Recursive Feature Elimination with Cross-Validation in Scikit Learn
In this article, we will earn how to implement recursive feature elimination with cross-validation using scikit learn package in Python. What is Recursive Feature Elimination (RFE)? Recursive Feature Elimination (RFE) is a feature selection algorithm that is used to select a subset of the most relev
5 min read
Creating Custom Cross-Validation Generators in Scikit-learn
Cross-validation is a fundamental technique in machine learning used to assess the performance and generalizability of models. Scikit-learn, a popular Python library, provides several built-in cross-validation methods, such as K-Fold, Stratified K-Fold, and Time Series Split. However, there are scen
6 min read
Cross validation in R without caret package
Cross-validation is a technique for evaluating the performance of a machine learning model by training it on a subset of the data and evaluating it on the remaining data. It is a useful method for estimating the performance of a model when you don't have a separate test set, or when you want to get
4 min read
Cross-validation on Digits Dataset in Scikit-learn
In this article, we will discuss cross-validation and its use on digit datasets. Further, we will see the code implementation using a digits dataset. What is Cross-Validation?Cross Validation on the Digits Dataset will allow us to choose the best parameters avoiding overfitting over the training dat
5 min read
Cross Validation in Machine Learning
Cross-validation is a technique used to check how well a machine learning model performs on unseen data. It splits the data into several parts, trains the model on some parts and tests it on the remaining part repeating this process multiple times. Finally the results from each validation step are a
7 min read
Cross-Validation Using K-Fold With Scikit-Learn
Cross-validation involves repeatedly splitting data into training and testing sets to evaluate the performance of a machine-learning model. One of the most commonly used cross-validation techniques is K-Fold Cross-Validation. In this article, we will explore the implementation of K-Fold Cross-Valida
12 min read
How to Deal with Factors with Rare Levels in Cross-Validation in R
Cross-validation is a vital technique for evaluating model performance in machine learning. However, traditional cross-validation approaches may lead to biased or unreliable results when dealing with factors (categorical variables) that contain rare levels. In this guide, we'll explore strategies fo
4 min read
Feature Selection in Python with Scikit-Learn
Feature selection is a crucial step in the machine learning pipeline. It involves selecting the most important features from your dataset to improve model performance and reduce computational cost. In this article, we will explore various techniques for feature selection in Python using the Scikit-L
4 min read
Cross Validation on a Dataset with Factors in R
Cross-validation is a widely used technique in machine learning and statistical modeling to assess how well a model generalizes to new data. When working with datasets containing factors (categorical variables), it's essential to handle them appropriately during cross-validation to ensure unbiased p
4 min read