Named Entity Recognition (NER) in NLP focuses on identifying and categorizing important information known as entities in text. These entities can be names of people, places, organizations, dates, etc. It helps in transforming unstructured text into structured information which helps in tasks like text summarization, knowledge graph creation and question answering.
Understanding Named Entity Recognition
NER helps in detecting specific information and sort it into predefined categories. It plays an important role in enhancing other NLP tasks like part-of-speech tagging and parsing. Examples of Common Entity Types:
- Person Names: Albert Einstein
- Organizations: GeeksforGeeks
- Locations: Paris
- Dates and Times: 5th May 2025
- Quantities and Percentages: 50%, $100
It helps in handling ambiguity by analyzing surrounding words, structure of sentence and the overall context to make the correct classification. It means context can change based on entity’s meaning.
Example 1:
- Amazon is expanding rapidly (Organization)
- The Amazon is the largest rainforest (Location)
Example 2:
- Jordan won the MVP award (Person)
- Jordan is a country in the Middle East (Location)
Working of Named Entity Recognition (NER)
Various steps involves in NER and are as follows:
- Analyzing the Text: It processes entire text to locate words or phrases that could represent entities.
- Finding Sentence Boundaries: It identifies starting and ending of sentences using punctuation and capitalization which helps in maintaining meaning and context of entities.
- Tokenizing and Part-of-Speech Tagging: Text is broken into tokens (words) and each token is tagged with its grammatical role which provides important clues for identifying entities.
- Entity Detection and Classification: Tokens or groups of tokens that match patterns of known entities are recognized and classified into predefined categories like Person, Organization, Location etc.
- Model Training and Refinement: Machine learning models are trained using labeled datasets and they improve over time by learning patterns and relationships between words.
- Adapting to New Contexts: A well-trained model can generalize to different languages, styles and unseen types of entities by learning from context.
Methods of Named Entity Recognition
There are different methods present in NER which are:
1. Lexicon Based Method
This method uses a dictionary of known entity names. This process involves checking if any of these words are present in a given text. However, this approach isn’t commonly used because it requires constant updating and careful maintenance of the dictionary to stay accurate and effective.
2. Rule Based Method
It uses a set of predefined rules which helps in extraction of information. These rules are based on patterns and context. Pattern-based rules focus on the structure and form of words helps in looking at their morphological patterns. On the other hand context-based rules focus on the surrounding words or the context in which a word appears within the text document. This combination of pattern-based and context-based rules increases the accuracy of information extraction in NER.
3. Machine Learning-Based Method
There are two main types of category in this:
- Multi-Class Classification: Trains model on labeled examples where each entity is categorized. In addition to labelling model also requires a deep understanding of context which makes it a challenging task for a simple machine learning algorithm.
- Conditional Random Field (CRF): It is implemented by both NLP Speech Tagger and NLTK. It is a probabilistic model that understands the sequence and context of words which helps in making entity prediction more accurate.
4. Deep Learning Based Method
- Word Embeddings: Captures the meaning of words in context.
- Automatic Learning: Deep models learn complex patterns without manual feature engineering.
- Higher Accuracy: Performs well on large varied datasets.
Implementation of NER in Python
Step 1: Installing Libraries
Firts we need to install necessary libraries. You can run the following commands in command prompt to install them.
!pip install spacy
!pip install nltk
!python -m spacy download en_core_web_sm
Step 2: Importing and Loading data
We will be using Pandas and Spacy libraries to implement this.
- nlp = spacy.load(“en_core_web_sm”): Loads the pre-trained “en_core_web_sm” SpaCy model and stores it in the variable nlp for text processing tasks.
Python import pandas as pd import spacy import requests from bs4 import BeautifulSoup nlp = spacy.load("en_core_web_sm") pd.set_option("display.max_rows", 200)
Step 3: Applying NER to a Sample Text
We have created some random content to implement this you can use any text based on your choice.
- doc = nlp(content): Processes text stored in content using the nlp model and stores resulting document object in the variable doc for further analysis.
- for ent in doc.ents: Iterates through the named entities (doc.ents) identified in the processed document and performs actions for each entity.
Python content = "Trinamool Congress leader Mahua Moitra has moved the Supreme Court against her expulsion from the Lok Sabha over the cash-for-query allegations against her. Moitra was ousted from the Parliament last week after the Ethics Committee of the Lok Sabha found her guilty of jeopardising national security by sharing her parliamentary portal's login credentials with businessman Darshan Hiranandani." doc = nlp(content) for ent in doc.ents: print(ent.text, ent.start_char, ent.end_char, ent.label_)
Output:
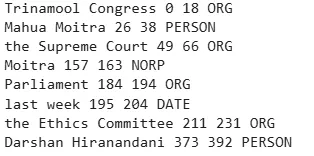
Resulting document
It displays the names of the entities, their start and end positions in the text and their predicted labels.
Step 4: Visualizing Entities
We will highlight the text with their categories using visualizing technique for better understanding.
- displacy.render(doc, style=”ent”): Visualizing named entities in the processed doc object by highlighting them in the text with their respective categories such as person, organization, location etc.
Python from spacy import displacy displacy.render(doc, style="ent")
Output:

Highlighted text with their categories
Step 5: Creating a DataFrame for Entities
- entities = [(ent.text, ent.label_, ent.lemma_) for ent in doc.ents]: Creating a list of tuples where each tuple contains the text, label (type) and lemma (base form) of each named entity found in the processed doc object.
Python entities = [(ent.text, ent.label_, ent.lemma_) for ent in doc.ents] df = pd.DataFrame(entities, columns=['text', 'type', 'lemma']) print(df)
Output:
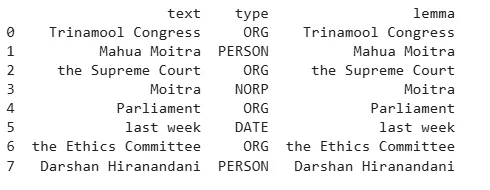
Text after categorization
Here dataframe provides a structured representation of the named entities, their types and lemmatized forms. NER helps organize unstructured text into structured information making it a useful for a wide range of NLP applications.
Similar Reads
Named Entity Recognition in NLP
In this article, we'll dive into the various concepts related to NER, explain the steps involved in the process, and understand it with some good examples. Named Entity Recognition (NER) is a critical component of Natural Language Processing (NLP) that has gained significant attention and research i
6 min read
Deep Face Recognition
DeepFace is the facial recognition system used by Facebook for tagging images. It was proposed by researchers at Facebook AI Research (FAIR) at the 2014 IEEE Computer Vision and Pattern Recognition Conference (CVPR). In modern face recognition there are 4 steps: DetectAlignRepresentClassify This app
8 min read
Human Activity Recognition with OpenCV
Have you ever wondered while watching a Sci-Fi film how does computer Recognize what a person's next move will be or how it predicts our actions according to activities performed? Well, the simple answer is it uses Human Activity Recognition (HAR) Technology for this. To accurately engineer features
8 min read
Applications of Pattern Recognition
Pattern recognition is the ability of a system to identify patterns and regularities in data by analyzing information. It helps systems to classify, cluster and interpret complex datasets, making it useful in fields like computer vision, healthcare, security and automation. In this article, we will
4 min read
Phoneme Recognition using Machine Learning
Phoneme recognition in Natural Language Processing (NLP) is a main component in developing speech recognition systems. Phonemes are the smallest sound units in a language that can distinguish one word from another. Recognising these phonemes accurately is important for converting spoken language int
7 min read
Convolutional Block for Image Recognition
INTRODUCTION: The basic neural network design i.e. an input layer, few dense layers, and an output layer does not work well for the image recognition system because objects can appear in lots of different places in an image. So the solution is to add one or more convolutional layers. A convolutional
5 min read
Image Recognition with Mobilenet
Introduction: Image Recognition plays an important role in many fields like medical disease analysis, and many more. In this article, we will mainly focus on how to Recognize the given image, what is being displayed. We are assuming to have a pre-knowledge of Tensorflow, Keras, Python, MachineLearni
5 min read
Types of Algorithms in Pattern Recognition
At the center of pattern recognition are various algorithms designed to process and classify data. These can be broadly classified into statistical, structural and neural network-based methods. Pattern recognition algorithms can be categorized as: Statistical Pattern Recognition â Based on probabili
5 min read
Audio Recognition in Tensorflow
This article discusses audio recognition and also covers an implementation of a simple audio recognizer in Python using the TensorFlow library which recognizes eight different words. Audio RecognitionAudio recognition comes under the automatic speech recognition (ASR) task which works on understandi
8 min read
Speech Recognition Module Python
Speech recognition, a field at the intersection of linguistics, computer science, and electrical engineering, aims at designing systems capable of recognizing and translating spoken language into text. Python, known for its simplicity and robust libraries, offers several modules to tackle speech rec
4 min read